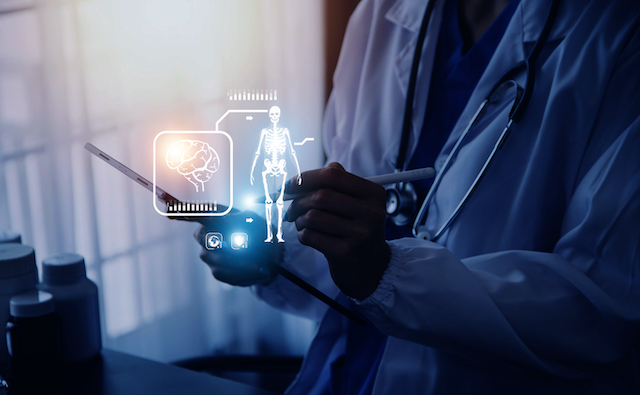
Pioneering AI tool developed by NHS teams to transform patient care
A ground-breaking artificial intelligence (AI) tool, designed to forecast patient health trajectories, has been developed by a collaboration of healthcare researchers within the NHS. Dubbed “Foresight,” this innovative tool is poised to revolutionise clinical decision-making processes, enhance monitoring in healthcare environments, and bolster clinical trials.
The development team is composed of specialists from two NHS foundation trusts in London—King’s College Hospital and Guy’s and St Thomas’—as well as academic experts from King’s College and University College London. Utilising the Cogstack platform, which is renowned for its capability in information retrieval and extraction, Foresight leverages natural language processing to efficiently mine data from NHS electronic health records. This allows the tool to be trained on vast amounts of healthcare data, employing a deep learning methodology to identify intricate patterns within both structured and unstructured data sources.
A critical evaluation published in The Lancet Digital Health illustrates Foresight’s effectiveness: the tool successfully predicted the next ten possible health disorders in a patient’s timeline with impressive accuracy rates—68% at King’s College Hospital, 76% at Maudsley NHS Foundation Trust, and an outstanding 88% with the US-based MIMIC-III dataset.
The tool’s utility was further underscored through a practical test where five clinicians created 34 hypothetical patient timelines based on simulated scenarios. An impressive 93% of the predictions made by Foresight were deemed clinically relevant, affirming their practical applicability in real-world settings.
According to a report from the NIHR Maudsley Biomedical Research Centre, Foresight’s capabilities are not limited to forecasting; it can also emulate clinical trials, facilitate longitudinal research, generate synthetic datasets, and simulate interventions to study disease progression. Professor Richard Dobson, a leading figure in medical informatics at King’s College London and the senior author of the study, expressed enthusiasm about the multitude of applications for Foresight. He highlighted its potential in creating digital health twins and advancing medical education, among other uses.
Professor Dobson emphasised the importance of employing high-quality data to refine AI models and expressed a vision for expanded collaboration. His aim is to involve more hospitals in the development of “Foresight 2,” an iteration that promises even greater accuracy through enhanced language models.
This initiative has garnered substantial support, receiving funding from the NHS AI Lab, the National Institute for Health and Care Research, and Health Data Research UK (HDRUK). Professor Andrew Morris, director of HDRUK, noted that the success of such innovations hinges on the quality and representativeness of the data used. He advocated for continued investment in the UK’s data infrastructure to ensure these advancements can be realised in a manner that is both secure and respectful of patient privacy.
Read More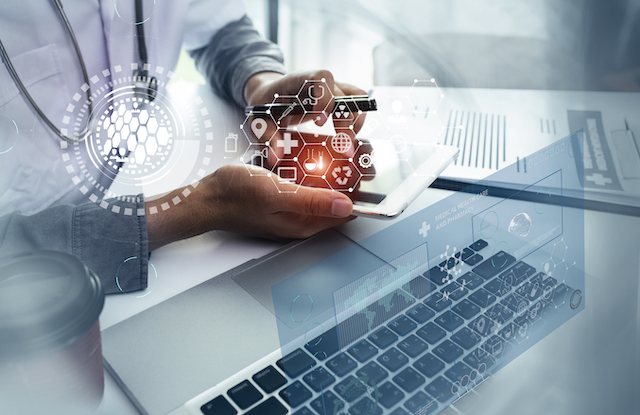
NHS adopts AI to combat absenteeism and expedite elective care waiting times
The National Health Service (NHS) is on the brink of an innovative leap, deploying artificial intelligence (AI) across an additional ten trusts with the aim of curtailing missed appointments and thus liberating valuable staff hours. This strategic move is anticipated to significantly dent the backlog in elective care waiting lists.
This initiative’s expansion comes in the wake of a triumphant pilot programme at the Mid and South Essex NHS Foundation Trust. This particular programme witnessed a near one-third reduction in patient no-shows within a mere six-month span.
The pioneering software, a collaborative creation between Deep Medical and contributions from both a frontline worker and an NHS clinical fellow, utilises algorithms alongside anonymised patient data to foresee potential appointment absences. It ingeniously taps into various external factors, such as weather conditions, traffic situations, and employment statuses, to deduce possible reasons behind a patient’s failure to attend. To counteract these challenges, it proposes alternative booking options that align more closely with the patient’s availability, including after-hours and weekend slots for those unable to take daytime leave.
Moreover, the software cleverly incorporates a system of intelligent backup bookings, ensuring that no clinical time goes to waste and optimising overall operational efficiency.
The six-month trial at the Mid and South Essex NHS Foundation Trust produced remarkable results: a 30% decrease in no-shows, 377 prevented missed appointments, and an additional 1,910 patients seen. Given these outcomes, projections suggest the trust could save approximately £27.5 million annually by persisting with this programme, benefiting a population of 1.2 million.
Deep Medical, under the co-founder duo Dr Benyamin Deldar and AI connoisseur David Hanbury, is at the forefront of this technological advancement. Dr Deldar highlights the software’s dual benefit: drastically reducing missed appointments and repurposing these slots for other patients, thereby enhancing both financial savings and public healthcare delivery.
The imminent roll-out to an additional ten trusts across England marks a significant step forward in this AI-powered journey.
In a concerted effort to recuperate elective care services post-pandemic and address prolonged waits for routine procedures, the NHS is embracing cutting-edge technologies and innovations, including AI. This approach aims to tackle the prevalent issue of missed hospital appointments, which amounts to hundreds of thousands each month, thus ensuring more judicious use of clinical time and expedited access to care for waiting list patients.
Annual statistics reveal a startling 6.4% no-show rate among the 124.5 million outpatient appointments across NHS England, translating into a financial strain of £1.2 billion.
The analysis also uncovers that physiotherapy appointments bear the brunt of absenteeism, with an 11% no-show rate, followed closely by cardiology, ophthalmology, and trauma and orthopaedics.
Dr Vin Diwakar, NHS England’s National Director for Transformation, praises the NHS’s innovative spirit and its openness to novel operational methods that ensure timely patient care. He underscores the AI initiative’s capacity to not only refine patient services but also to foster a more economical utilisation of taxpayer funds.
Moreover, he emphasises the role of such AI pilots in empowering patients to manage their healthcare more effectively and in addressing health inequalities.
The University Hospitals Coventry and Warwickshire (UHCW) NHS Trust exemplifies another facet of AI application through ‘process mining’. This technique offers insights into the efficacy of existing processes, spotlighting bottlenecks and areas ripe for improvement.
Notably, during its pilot, the Trust identified a correlation between high deprivation scores and increased DNAs, with a marked surge in last-minute cancellations post two SMS reminders. Adapting their strategy to send reminders 14 days and then four days prior to an appointment significantly reduced no-show rates from 10% to 4% among a targeted patient group.
Encouraged by these results, the Trust is now exploring the application of process mining to theatre scheduling, aiming for further efficiency gains and enhancements
Read More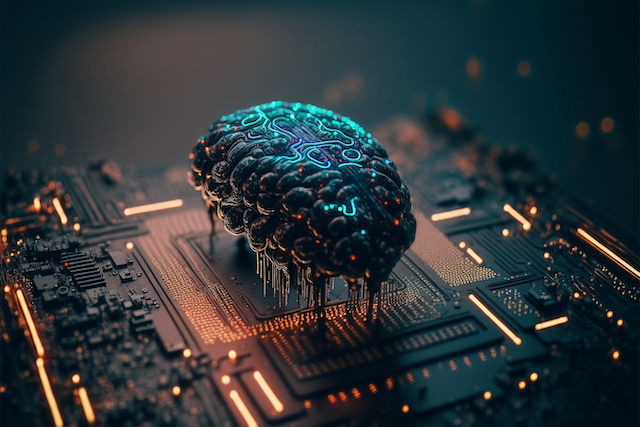
Mayo Clinic teams up with Cerebras Systems to advance healthcare AI
The Mayo Clinic, a renowned non-profit medical institution based in Rochester, Minnesota, announced a strategic partnership with the Silicon Valley-based startup Cerebras Systems on Monday (15th of January, 2024). This collaboration aims to harness the power of artificial intelligence (AI) in enhancing healthcare services.
With significant presences across three major campuses in the United States and additional facilities in the United Kingdom and the United Arab Emirates, the Mayo Clinic is set to utilise cutting-edge computing chips and systems supplied by Cerebras. The collaboration will delve into the Mayo Clinic’s extensive archive of anonymised medical records and data, laying the groundwork for the development of bespoke AI models.
According to Matthew Callstrom, Mayo’s Medical Director for Strategy and Chair of the Radiology Department, these AI models are poised to revolutionise various aspects of medical record management and diagnostics. Some models are being designed to interpret and summarise extensive medical records for new patients, streamlining the patient onboarding process. Additionally, other models will focus on identifying intricate patterns in medical imagery and genome data, patterns that might elude even the most experienced medical professionals. However, Callstrom emphasised that these systems are intended to augment medical decision-making, not replace it. The human expertise of doctors remains paramount in the clinical decision-making process.
“The integration of AI is about enhancing the decision-making process for each patient, considering the multitude of factors and drawing upon extensive experience,” Callstrom explained.
The Mayo Clinic’s collaboration with Cerebras is anticipated to yield results that will be accessible via the Mayo Clinic Platform. This platform is a comprehensive data network already utilised by various healthcare systems such as Mercy in the U.S., the University Health Network in Canada, and other systems in Brazil and Israel.
While the pricing model for the AI technology developed through this partnership has not been finalised, Callstrom indicated that the Mayo Clinic intends to disclose further details about this venture during a presentation at JPMorgan Chase’s healthcare conference in San Francisco.
Andrew Feldman, CEO of Cerebras, described the deal as a multi-million-dollar agreement spanning several years, though he refrained from disclosing specific financial details. Cerebras, aspiring to compete with industry leaders like Nvidia, will provide both the necessary hardware and software development expertise as part of the agreement. This collaboration marks a significant step in integrating advanced AI capabilities into the healthcare sector, aiming to enhance patient care through technological innovation.
Read More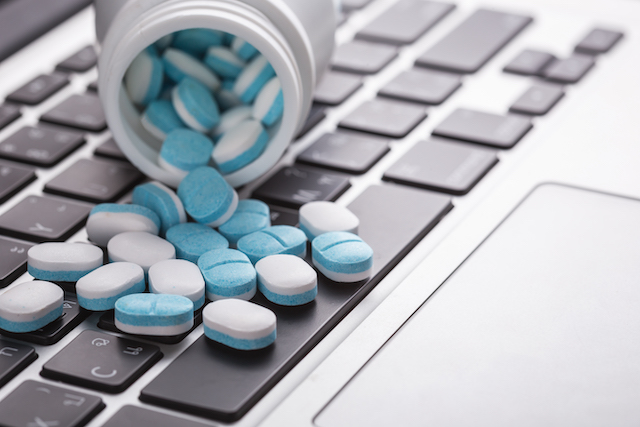
New machine learning approach transforms behavioural health medication practices
At the recent AMCP Nexus 2023 conference in Orlando, Florida (16th-19th of October), presenters showcased a groundbreaking machine learning program designed to address medication-related issues in individuals with behavioural health conditions. This program has shown promising results in reducing polypharmacy, enhancing medication adherence, and decreasing healthcare costs.
Behavioural health conditions pose a significant challenge to healthcare systems. A 2020 Milliman study examining commercial healthcare claims data from 2017, which encompassed 21 million people, revealed that although only 27% had a behavioural health condition, they accounted for over half of the total healthcare expenditures. In this context, machine learning offers a potential solution for better managing these conditions.
The presenters highlighted the issue of polypharmacy, a common concern in behavioural health where 60% of adults with a condition are prescribed two or more psychotropic medications. Polypharmacy not only increases the risk of drug interactions and adverse events but also contributes to soaring healthcare costs. Dr. Caroline Carney, Chief Medical Officer at Magellan Health, underscored the tendency for medication overlap and overprescription in treating conditions like depression and anxiety, often leading to unnecessary medication layers.
Another issue in managing behavioural health medications is the multiple prescribers involved, including primary care doctors, specialists, and inpatient clinicians, often resulting in uncoordinated treatment. This lack of coordination can leave patients confused and overwhelmed with their medication regimens.
To combat these challenges, Magellan Health collaborated with Arine, a medication management tech startup, to create inforMED (formerly known as Navigate Whole Health). This AI-driven program identifies prescribers who can potentially optimise patient care, generating comprehensive care plans with treatment recommendations and patient education. The program’s effectiveness is continuously improved by incorporating new clinical outcome data.
Dr. Carney elaborated on the program’s approach, which considers hundreds of parameters, providing prescribers not just with medication change suggestions but also with reasons, implications, and evidence-based support for the recommended changes.
Yoona Kim, PharmD, PhD, co-founder and CEO of Arine, explained that machine learning algorithms are utilised to target prescribers based on their prescribing patterns and the presence of prescribing outliers in their patient panels. The program also considers social determinants of health, using ZIP code data to assess potential barriers to healthcare access, such as low income or lack of vehicle access.
The results of this program have been significant. Dr. Kim reported a reduction in behavioural health polypharmacy by 45% to 55%, a 20% increase in medication adherence, a 20% reduction in average daily morphine milligram equivalents, and a savings of $360 to $840 in pharmaceutical costs per enrolled member annually.
Dr. Carney emphasised the program’s success in providing actionable data and guidance to healthcare providers, leading to improved patient outcomes and stronger, longer-lasting professional relationships. This innovative approach signifies a major step forward in the management of medication for behavioural health conditions.
Read More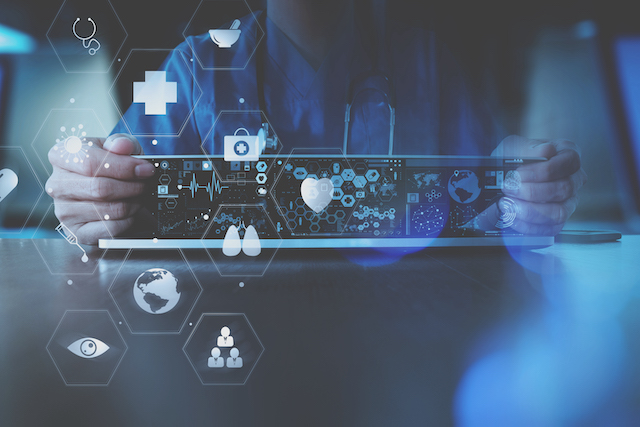
NHS England’s controversial £330m data deal with Palantir draws mixed reactions
NHS England’s recent decision to award the £330 million Federated Data Platform (FDP) contract to US data analytics firm Palantir, in collaboration with Accenture, PwC, NECS, and Carnall Farrar, has elicited a spectrum of reactions. This announcement marks a significant step in NHS’s digital transformation efforts, with the FDP designed to connect and streamline access to healthcare data across the NHS.
Matthew Taylor, CEO of the NHS Confederation, acknowledged the FDP’s potential in enhancing care delivery by freeing up clinical time and fostering efficient, safer patient care. However, he emphasised the need for substantial efforts to garner public support for the initiative.
Contrasting opinions emerged from within the NHS. Paul Jones, Chair of the Digital Health Networks CIO Advisory Panel, expressed disappointment at NHS England’s decision to proceed despite reservations from trust digital teams and the financial constraints on other NHS digital budgets.
Nick Wilson, CEO at System C, recognised the wealth of experience within the NHS and technology companies in digital transformation but voiced disappointment over the contract not being awarded to a British consortium. He also highlighted concerns about the exclusion of GP data from the FDP, stressing the complexities of interoperability in health and social care and urging Palantir and Accenture to learn from past challenges.
The decision faced criticism from those who had campaigned against Palantir’s involvement. The Good Law Project, a not-for-profit campaign organisation, is preparing legal challenges to ensure proper handling of sensitive NHS data, focusing on maintaining privacy.
Cori Crider, Director of Foxglove, a legal advocacy organisation, raised questions about the FDP’s effectiveness, citing unsuccessful hospital trials of Palantir’s technology. Dr David Nicholl, spokesperson for Doctors’ Association UK, echoed these concerns, questioning the scrutiny around the deal and the preliminary results of NHS trials with Palantir’s technology.
David Davis, MP for Haltemprice and Howden, expressed reservations about Palantir’s suitability for handling sensitive data, citing their background in espionage and concerns about data protection.
In response to these varied perspectives, NHS England has garnered support from several organisations, including the NHS Confederation, National Voices, and the Academy of Royal Medical Colleges. National Voices CEO Jacob Lant and Dr Jeanette Dickson, Chair of the Academy of Medical Royal Colleges, acknowledged the FDP’s potential in driving digital innovation and enhancing data connectivity in the NHS.
To address public concerns, NHS England is developing an engagement portal for the public to learn more about the FDP and submit queries. Additionally, Dr Nicola Byrne, National Data Guardian, and Dr Nicola Perrin of the Association of Medical Research Charities have joined the independent Check and Challenge Group for the FDP, overseen by NHS England.
Furthermore, NHS England has assured that data sharing under the FDP will not commence until new ‘Privacy Enhancing Technologies’ (PET) are developed and implemented, expected by April 2024. Details on these technologies, being developed by a separate supplier, are anticipated to be released later in the year.
Read More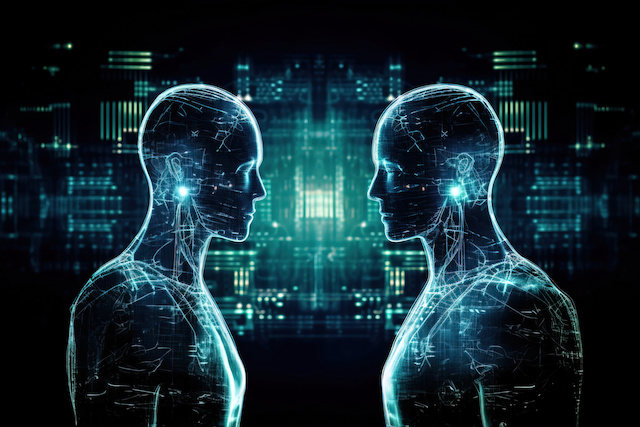
Personalised healthcare revolution: The rise of digital twins in medicine
The trajectory of medicine is being redefined by pioneering research into computational models, advancing towards a future where medical treatments are tailored not to the average patient, but to each individual. Envision possessing a ‘digital twin’—a virtual counterpart that can undergo trials and treatments, sparing you the need for direct medication or surgical intervention. Scientists project that within the next decade, we could witness the routine use of ‘in silico’ trials, utilising virtual organs to evaluate drug safety and effectiveness, while bespoke organ models might be employed to customise patient care and avert medical complications.
Digital twins represent sophisticated computer-generated replicas of physical entities or processes, continuously refined with data from their actual counterparts. In the medical realm, this entails the fusion of extensive biological data—including genetic, proteomic, cellular, and systemic information—with individual patient data to craft detailed virtual models of their organs, and potentially, in time, their entire body.
Professor Peter Coveney, Director of the Centre for Computational Science at University College London and co-author of ‘Virtual You’, suggests that much of current medical practice lacks a scientific underpinning. He compares it to navigating by looking in the rear-view mirror—basing treatment for the patient at hand on historical cases. “A digital twin utilises your own data within a model that encapsulates your unique physiology and pathology. It’s a move away from decisions based on potentially unrepresentative population data to truly personalised medicine,” explains Prof. Coveney.
Cardiology is at the forefront of this cutting-edge model. Companies are already harnessing patient-specific heart models to aid in the design of medical devices. Meanwhile, the Barcelona-based enterprise ELEM BioTech is at the forefront, granting companies the capability to test drugs and devices on simulated human hearts. “We’ve conducted numerous virtual human trials on several compounds and are on the cusp of launching a new phase, with our cloud-ready product accessible to pharmaceutical clients,” shares Chris Morton, co-founder and CEO of ELEM.
At the recent Digital Twins conference hosted by the Royal Society of Medicine in London, Dr. Caroline Roney from Queen Mary University of London detailed the development of tailored heart models which could significantly aid surgeons in planning interventions for atrial fibrillation patients. “Surgeons typically resort to average-based approaches, but crafting patient-specific predictions that forecast long-term outcomes remains a formidable challenge,” Dr. Roney stated. She foresees widespread application of this technology in cardiovascular treatments, including decisions on valve selection and placement during replacements.
The field of oncology is also poised to benefit from digital twins. Teams from GSK and King’s College London are joining forces to construct virtual duplicates of patient tumours, amalgamating imaging, genetic, and molecular data with 3D cultures of cancer cells, and observing their drug responses. Leveraging machine learning, researchers can foresee how individual patients may react to various treatments, drug combinations, and dosages. “Conducting repetitive trials on a real patient with multiple treatments isn’t viable. Our aim is to devise a strategy while the patient is still with us, preparing us for any recurrence of cancer,” said Professor Tony Ng from King’s College.
The advent of digital twins extends even to the realm of pregnancy, offering the potential to develop treatments for conditions such as placental insufficiency or pre-eclampsia, and deepening our grasp of pregnancy and labour physiology. Professor Michelle Oyen, Director of the Center for Women’s Health Engineering at Washington University in St Louis, is crafting placenta models from ultrasound scans and post-birth high-resolution imagery to predict complications during pregnancy. “We’re striving to identify measures in a live person that could forewarn us of placental issues, aiming to preempt adverse outcomes like stillbirth,” Prof. Oyen elucidates.
In collaboration, Professor Kristin Myers from Columbia University is modelling the cervix, uterus, and foetal membranes, with the overarching goal to merge these into a comprehensive individual model to predict pregnancy outcomes. “We hope to analyse a simple ultrasound scan to understand how the uterus will adapt and when labour might occur,” Prof. Myers aspires, potentially guiding decisions on interventions like caesarean sections.
Moreover, the concept of digital twins is being expanded to model entire hospitals to enhance patient flow and healthcare system efficiency. Dr. Jacob Koris, a trauma and orthopaedic surgeon and digital lead at Getting It Right First Time, describes how tracking digital footprints left by patient interactions—from X-rays to outpatient appointments—can provide a granular, real-time view of patient treatment pathways. “Such insights could pinpoint areas for improvement and exemplary practices that could revolutionise patient care,” Dr. Koris believes.
This ambitious step forward in computational medicine promises a leap from the traditional, one-size-fits-all model to a future where every treatment is as unique as the patient it serves.
Read More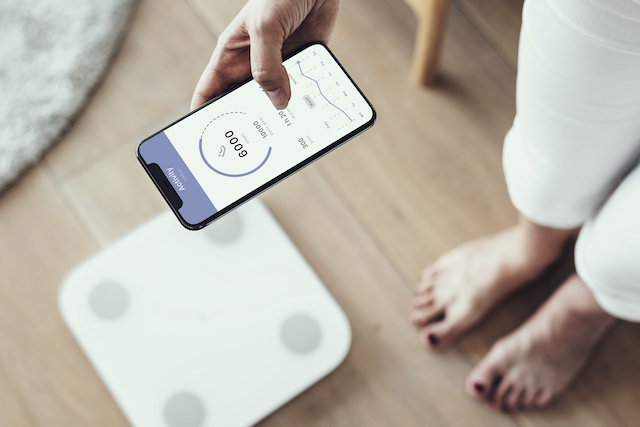
Digital tool for specialist weight management services shows promising results
In a groundbreaking investigation led by the team at University Hospitals Coventry and Warwickshire NHS Trust (UHCW), the potential and efficacy of digital platforms tailored for advanced weight management have been highlighted.
The research revolved around assessing the eagerness, acceptance, and active participation of patients on standby for their first-ever specialist weight management consultation. In the United Kingdom, specialised weight management services, often referred to as tier 3 services, offer a holistic approach to tackling obesity. These specialised services are generally anchored in hospitals or clinic facilities and bring together a diverse group of healthcare specialists. This includes dietitians, psychologists, specialist nurses, and doctors, all proficient in the realm of weight management.
For the purposes of this research, an NHS-approved digital platform named Gro Health was integrated into the service offering. This avant-garde health application propels numerous healthcare routes, with its tier 3 weight management feature, “W8Buddy”, acting as an online weight loss clinic. This feature delivers structured learning sessions, both individual and group coaching, an expansive list of over 2,000 recipes and meal schedules, and tools for health and nutrition tracking to chart progress.
The study drew in 199 prospective patients eagerly waiting for their appointment at the NHS Trust’s tier 3 weight management service.
Preliminary results indicate that over half of these individuals expressed genuine interest in the application. An impressive one-third went on to actively engage with the digital platform, highlighting the immense potential of such digital interventions in the specialised weight management scenario.
The engagement analysis unearthed intriguing data points. Those prone to emotional eating or those with an escalated BMI exhibited an increased propensity towards the Gro Health application. Meanwhile, aspects like age, ethnic background, and metabolic indicators like glycemia and lipid readings did not notably sway the interest.
These findings could serve as a blueprint for revolutionising weight management strategy. As digital healthcare tools evolve and gain traction, they stand poised as formidable and expansive strategies to confront the global issue of obesity.
Charlotte Summers, a behavioural change expert and the Founding Chief Operations Officer, expressed her enthusiasm, noting, “The pronounced interest demonstrated by patients in the Gro Health W8Buddy tool for weight management is truly heartening.”
She drew attention to the evident link between emotional eating, a raised BMI, and heightened engagement, highlighting, “This relationship underscores the transformative capacity of precise digital strategies in addressing weight-related concerns.”
Summers further elaborated on the journey ahead, “As we venture into providing tier 3 and 4 weight management services, we’re thrilled about tailoring these platforms with firsthand insights from both patients and healthcare providers. Such a collaborative effort not only champions a patient-driven model but also deepens our grasp on their preferences and anticipations. This, in turn, empowers us to offer top-tier, accurate care, be it through enhancing conventional healthcare avenues or pioneering virtual healthcare experiences.”
The study’s authors stress the need for continued exploration into understanding the challenges and motivators behind adopting digital tools and emphasise the importance of rigorously assessing their impact within specialised weight management services.
The rise of digital health platforms is sculpting the future of healthcare. This specific investigation underscores the transformative power of such tools, all while highlighting the necessity to unravel the complexities of patient engagement. As we witness the proliferation and capabilities of digital health platforms, the persistent quest to maximise their utility for patients and the broader healthcare spectrum is paramount.
Stay tuned for more revelations as ongoing studies continue to sculpt this rapidly evolving domain of weight management.
Read More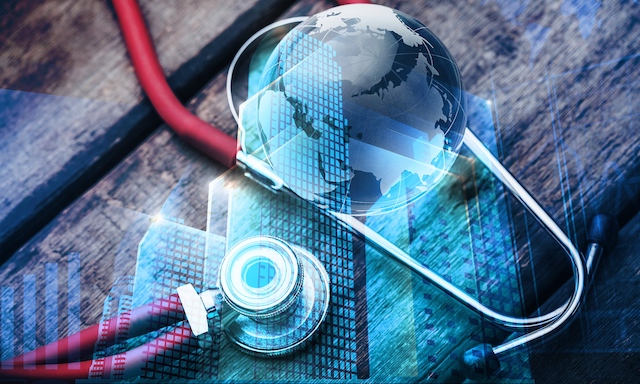
WHO Europe stresses the need for strengthened digital health literacy across the region
In a significant report released by WHO Europe, there’s an emphasised call for an immediate boost in investments towards digital health literacy across the region.
The report, titled “Digital Health in the European Region: The Ongoing Commitment to Transformation,” sheds light on a concerning statistic: only half of the countries spanning Europe and Central Asia have rolled out policies tailored to bolster digital health literacy. This oversight leaves a considerable portion of the population in the shadows, devoid of the benefits of evolving digital healthcare platforms.
The landscape of healthcare has experienced a monumental shift in the WHO European Region over recent times, as is evident from the burgeoning adoption rate of digital health solutions. This transformation has redefined the dynamics of patient care.
Released during the Second WHO Symposium on the Future of Health Systems in a Digital Era held for the European Region, the report encompasses insights from all 53 member nations. Many countries within this bracket witnessed a spontaneous surge in the creation and deployment of digital health tools and policies due to the pressing demands of the COVID-19 pandemic – a time dominated by lockdowns and social distancing mandates. This led to the broader acceptance of telemedicine services and the advent of user-centric health applications. However, the report firmly stresses that the journey is far from complete.
A pressing concern is the evident disparity in the adoption and assimilation of digital health solutions across the region. This “digital health divide” implies that a staggering number of individuals are yet to harness the potential benefits of digital health advancements.
Diving deeper, the report draws attention to several pivotal areas:
- Of the countries surveyed, 44 have an established national digital health strategy.
- A unanimous consensus is seen with all 53 member nations having legislation that focuses on protecting individual data privacy.
- However, there’s a considerable disparity in the preparedness and execution, with only 19 countries offering guidance on evaluating the efficacy and safety of digital health initiatives.
- Slightly above 50% of these countries have put forth policies advocating digital health literacy and have set into motion a digital inclusion agenda.
- The pandemic saw 30 countries devising legislation to champion the cause of telehealth.
- An area that requires immediate attention is the oversight of mobile health (mHealth) applications. Many nations lack a dedicated body to ensure the quality, safety, and reliability of these apps. A mere 15% have reported systematic evaluations of state-backed mHealth initiatives.
- Just above half of the countries have strategised the application of Big Data and avant-garde analytics within the healthcare domain.
Dr. Hans Henri P. Kluge, the WHO Regional Director for Europe, opined on the matter, “In numerous nations, the growth trajectory of digital health platforms has been somewhat sporadic. This approach warrants an overhaul. It’s imperative to perceive digital health as a long-term, strategic investment, rather than a fleeting addition or a privilege enjoyed by a select few. It is a clarion call for our political and health leaders to strategically invest in the digital health infrastructure of tomorrow, today.”
Read More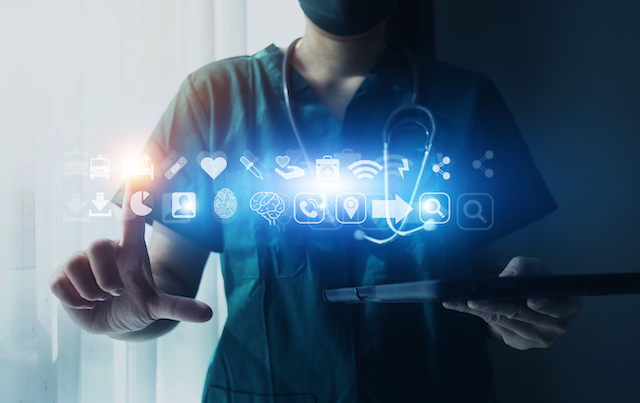
NHS gets £30m boost for tech advancements to improve patient care
In a recent announcement, the Department of Health and Social Care (DHSC) revealed its plans to allocate £30 million for state-of-the-art technology aimed at enhancing services provided by the NHS. This funding is anticipated to play a pivotal role in reducing patient wait times, expediting the diagnostic process, and introducing novel patient treatment methodologies.
The DHSC, on its website, highlighted that such financial backing is expected to alleviate some of the operational burdens the NHS might face during the upcoming winter season. Notably, the funds could potentially be utilised to expand 3D diagnostics, thus expediting cancer screenings, and to implement innovative logistic solutions such as drone deliveries.
Moreover, another significant avenue the investment could support is the augmentation of virtual wards. This would allow more patients to receive essential care within the comfort of their homes, ensuring hospital beds remain available for those in acute need. To date, the NHS has successfully established over 9,800 virtual ward beds, with plans to achieve the 10,000 bed milestone before winter strikes.
Regions throughout England can access this funding. Integrated care systems (ICSs) have been tasked with submitting proposals to both the DHSC and NHSE detailing how they would best leverage the technology. The application process is set to commence shortly.
Health and Social Care Secretary, Steve Barclay, emphasised the government’s commitment to ensuring the medical fraternity is equipped with cutting-edge technology, stating, “From virtual ward beds to wearable medical devices, this new funding is a testament to our dedication to enhancing patient care, preparing for winter, and relieving hospital pressures.”
In addition to supporting the use of wearable devices that monitor vital signs and aid in the management of chronic ailments, ICSs might channel investments into advanced digital imaging, a move that would undoubtedly bolster diagnostic capabilities, especially in the realms of cancer detection and other severe illnesses.
Dr Vin Diwakar, NHS’s interim national director of transformation, applauded the NHS’s innovative prowess, stating that such tech advancements have already positively impacted over 210,000 patients through virtual ward setups. Ellie Kearney, a spokesperson from the Health Tech Alliance, welcomed the financial boost but also expressed some members’ discontent with certain previous funding strategies.
In further developments, the DHSC referenced the Medical Technology Strategy they unveiled earlier in the year, which laid down a roadmap for enabling patient access to secure, efficient, and pioneering tech via the NHS. This latest £30 million injection builds upon a prior £21 million allocation towards AI diagnostic tools.
This strategic funding alignment is in sync with the government’s overarching vision for fortifying the NHS, especially with the challenges that winter typically brings. In addition to this tech fund, the government, in September, infused £200 million into the NHS, aiming to fortify its resilience. The Urgent and Emergency Care Recovery Plan, rolled out at the beginning of the year, pledges to furnish 5,000 more hospital beds, 10,000 virtual ward beds, and 800 brand-new ambulances, supported by an impressive £1 billion fund.
Read More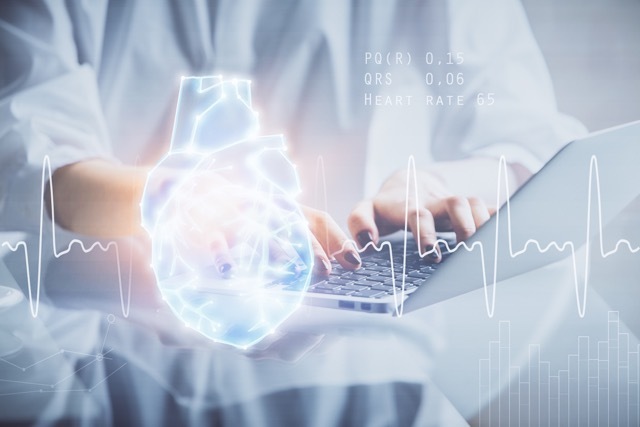
Public support key to leveraging UK’s healthcare data, research suggests
The latest research from the Boston Consulting Group Centre for Growth underscores the potential held by healthcare data in the UK. The report, titled ‘Towards a healthier, wealthier UK: unlocking the value of healthcare data‘, highlights robust public support for optimising the UK’s healthcare data, emphasising its dual potential to revitalise the economy and alleviate strains on the healthcare system.
Historically, the concept of harnessing healthcare data has been proposed numerous times in the UK. Still, its full potential remains largely untapped. According to the Boston Consulting Group, the roadmap to actualising this vision rests on two pillars: gaining comprehensive public endorsement for healthcare data utilisation and redirecting its generated value back into the healthcare sector.
Empirical evidence has demonstrated the tangible benefits of leveraging healthcare data. A standout case is the Royal Free London NHS Foundation Trust, which managed to curtail £2,000 in hospital admissions for acute kidney injury patients. Their success was anchored on an innovative app which synthesised data from blood tests, medical archives, and clinical assessment tools to provide timely alerts to healthcare professionals upon identifying potential risks.
An exemplary initiative from the Netherlands further fortifies this narrative. A consortium of seven academic hospitals implemented a value-driven healthcare model, leading to a remarkable 30% decline in unnecessary patient admissions and a staggering 74% drop in follow-up surgeries due to complications. This was achieved by pinpointing the right metrics to enhance patient outcomes and fostering a culture of data-sharing across institutions.
Lord O’Shaughnessy, a luminary from Newmarket Strategy and ex-parliamentary undersecretary of state at the Department of Health, opined, “Properly harnessed healthcare data has the potential to amplify both the UK’s health standards and economic vigour. However, this transformation hinges on unshakeable public trust. Establishing this trust mandates a rigorous, inclusive, and adaptive mechanism for public engagement and decision-making.”
Contrary to prevalent misconceptions, the Boston Consulting Group’s report revealed that a staggering 90% of individuals are amenable to sharing their health data with the NHS, reiterating that this openness is contingent upon the intended use of this data. While 73% endorsed the use of their data to gauge potential health risks, 72% favoured its application in refining clinical care methodologies.
The public’s fervent desire to be privy to discussions concerning their health data’s usage was palpable. In London, this dialogue has already gained momentum with the OneLondon Citizen Advisory Group finalising recommendations for the city’s Health Data Strategy.
On the matter of monetising healthcare data, most respondents were comfortable with their data being profitable, provided a portion of the proceeds revitalises the healthcare sector or catalyses broader societal benefits.
Key Recommendations:
In its concluding remarks, the Boston Consulting Group’s report delineated actionable steps to actualise the potential of healthcare data:
- Showcase the tangible benefits derived from accessible healthcare data to foster public comprehension and endorsement.
- Orchestrating a cohesive public engagement drive across all NHS data-centric ventures, elucidating the tangible benefits of optimised data usage.
- Involve the public in data-related decision-making through decision panels and data usage logs, ensuring they remain integral stakeholders in shaping the discourse.
- Establish a central reservoir to channel the monetary value extracted from data into local NHS frameworks.
Concluding his thoughts, Lord O’Shaughnessy urged policymakers to champion a grand public outreach initiative around healthcare data, stating, “Without engaging the masses in this crucial dialogue, we stand at the precipice of forfeiting an unprecedented opportunity.”
Read More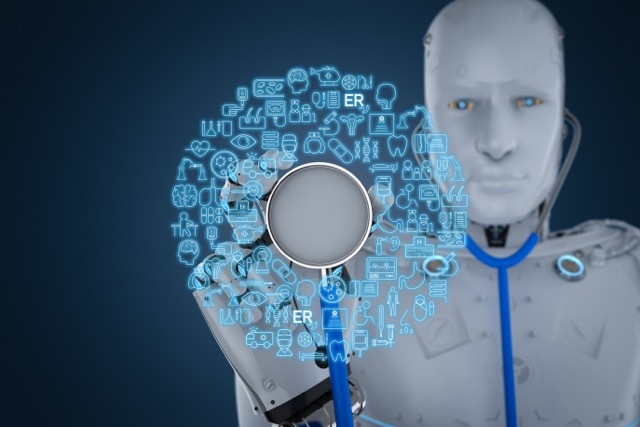
Revolutionary AI tool forecasts pancreatic cancer risk up to three years in advance
Ground-breaking research spearheaded by Harvard Medical School, in collaboration with the University of Copenhagen, VA Boston Healthcare System, Dana-Farber Cancer Institute, and the Harvard T.H. Chan School of Public Health, has developed an artificial intelligence (AI) instrument capable of identifying individuals at the greatest risk of developing pancreatic cancer up to three years before diagnosis, using solely their medical records.
The study, published in Nature Medicine on May 8, indicates that implementing AI-driven population screening could be a key strategy in detecting those at a high risk of pancreatic cancer earlier. This could, in turn, hasten the diagnosis of a condition often detected at advanced stages when treatment options are less effective, resulting in poorer outcomes. Pancreatic cancer, one of the world’s deadliest malignancies, is anticipated to increase its mortality toll.
At present, there is an absence of population-wide screening tools for pancreatic cancer. Targeted screenings are performed for individuals with certain genetic mutations or a family history that increases their risk of developing the disease. However, these screenings may overlook other cases not fitting these criteria, the researchers highlighted.
The study’s co-senior investigator, Chris Sander, a faculty member in the Department of Systems Biology at the Blavatnik Institute at HMS, underscored the significance of the AI tool. “Deciding who is at a high risk for a disease and would benefit from additional testing is one of the most challenging determinations clinicians have to make. The tests can be more invasive, more costly, and carry their own risks. An AI tool that accurately identifies those at the highest risk for pancreatic cancer and who would gain the most from additional tests could greatly enhance clinical decision-making.”
If implemented widely, this AI-driven method could expedite the detection of pancreatic cancer, lead to earlier treatment, and improve patient outcomes, possibly extending their life spans.
“AI-driven screening provides the opportunity to change the course of pancreatic cancer, a formidable disease that is exceptionally challenging to diagnose early and treat promptly,” said study co-senior investigator Søren Brunak, a professor of disease systems biology and research director at the Novo Nordisk Foundation Center for Protein Research at the University of Copenhagen.
In this novel study, the researchers trained the AI algorithm on two separate data sets, containing a total of 9 million patient records from Denmark and the United States. They instructed the AI model to identify potential signs of pancreatic cancer risk based on the data in the records.
The model could predict patients likely to develop pancreatic cancer in the future by identifying combinations of disease codes and the timing of their occurrence. Interestingly, many of the symptoms and disease codes were not directly related to or derived from the pancreas.
The researchers evaluated different versions of the AI models for their capacity to identify individuals at a heightened risk of disease development over different timescales – 6 months, one year, two years, and three years.
Overall, each iteration of the AI algorithm proved considerably more precise in predicting who would develop pancreatic cancer than current estimates of disease incidence in the general population. The researchers proposed that the model is likely as accurate in predicting disease onset as the existing genetic sequencing tests, which are generally only accessible to a small subset of patients in data sets.
Screening techniques for certain prevalent cancers, such as breast, cervix, and prostate cancer, rely on relatively straightforward and highly effective techniques, such as a mammogram, a Pap smear, and a blood test. These methods have significantly improved the outcomes for these diseases by ensuring early detection and intervention.
In contrast, pancreatic cancer poses greater challenges and costs in terms of screening and testing. Doctors predominantly focus on family history and the presence of genetic mutations. While these are crucial indicators of future risk, they often overlook many patients.
The AI tool presents a significant advantage in its potential applicability to any patient for whom health records and medical history are available, not solely those with a known family history or genetic predisposition for the disease. This is particularly important, the researchers noted, because many patients at a high risk may not be aware of their genetic predisposition or family history.
In the absence of clear indications that a person is at high risk for pancreatic cancer and without symptoms, clinicians may understandably hesitate to recommend more sophisticated and costlier testing methods such as CT scans, MRI, or endoscopic ultrasound.
When these tests are performed and suspicious lesions are detected, the patient must undergo a procedure to obtain a biopsy. Given its deep placement in the abdomen, the pancreas is difficult to reach and easy to inflame, leading to its nickname as “the angry organ.”
The researchers advocate for an AI tool that singles out those at the greatest risk for pancreatic cancer. This would ensure clinicians are testing the correct population, while also preventing others from undergoing unnecessary testing and additional procedures.
The survival rate for those diagnosed with pancreatic cancer in its early stages is about 44 percent, five years post-diagnosis. However, only 12 percent of cases are diagnosed at this stage. The survival rate decreases dramatically to 2 to 9 percent for those with tumours that have spread beyond their origin, the researchers estimated.
Chris Sander emphasised, “Despite significant advancements in surgical techniques, chemotherapy, and immunotherapy, the survival rate remains low. Therefore, besides advanced treatments, there’s a pressing need for better screening, more focused testing, and earlier diagnosis. This is where the AI-based approach serves as the initial critical step in this process.”
For the current study, the researchers created multiple versions of the AI model and trained them on the health records of 6.2 million patients from Denmark’s national health system over a 41-year span. Of these patients, 23,985 developed pancreatic cancer over time.
During the training, the algorithm identified patterns suggesting future pancreatic cancer risk based on disease trajectories. For instance, diagnoses such as gallstones, anaemia, type 2 diabetes, and other gastrointestinal-related issues pointed to a higher risk for pancreatic cancer within three years of evaluation.
Inflammation of the pancreas was a strong predictor of future pancreatic cancer within an even shorter time span of two years.
The researchers caution that none of these diagnoses on their own should be deemed indicative or causative of future pancreatic cancer. However, the pattern and sequence in which they occur over time provide clues for an AI-based surveillance model and could prompt physicians to closely monitor or test those at elevated risk.
Next, the researchers tested the best-performing algorithm on an entirely new set of patient records it had not previously seen — a U.S. Veterans Health Administration data set comprising nearly 3 million records over 21 years, including 3,864 individuals diagnosed with pancreatic cancer.
The tool’s predictive accuracy was somewhat lower on the US data set. The researchers attributed this to the shorter collection period and the different patient population profiles in the U.S. dataset compared to the Danish dataset.
When the algorithm was retrained from scratch on the U.S. dataset, its predictive accuracy improved. This, the researchers said, underscores the importance of training AI models on high quality, rich data and the necessity of access to large representative datasets of clinical records aggregated nationally and internationally.
In the absence of globally valid models, AI models should be trained on local health data to ensure their training reflects the specific characteristics of local populations.
Read More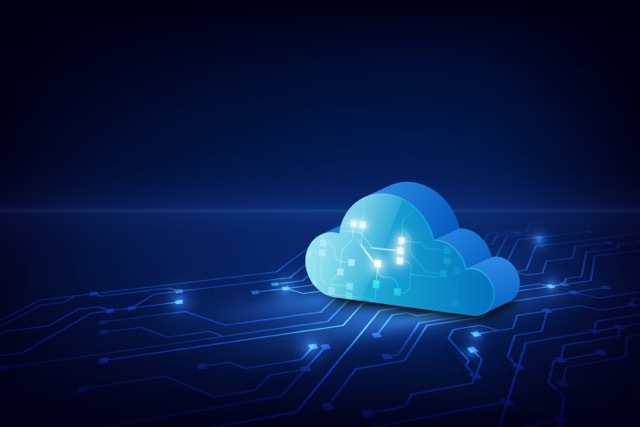
New report illuminates the promising role of cloud technology in healthcare
A fresh report spotlighting the transformative role of cloud technology in combating the prevalent challenges in healthcare has been released by the Health Policy Partnership. The publication, titled “Our Health in the Cloud: Exploring the Evolving Role of Cloud Technology in Healthcare,” outlines the practical application of this technology. The report was created with the support of the European Institute for Innovation through Health Data (i~HD).
The publication coincides with the HIMSS 2023 European Health Conference & Exhibition in Lisbon, and delves into how cloud technology has propelled innovative solutions within the healthcare industry, and its potential future contributions.
Dipak Kalra, president of the i~HD, commented, “Cloud technology is a crucial catalyst for leveraging health data to enhance health outcomes, boost patient safety, swiftly identify public health threats, and expedite research into novel medicines and medical technologies.”
“Cloud technology offers secure computational power surpassing conventional on-premise resources, facilitating data integration across entities. Our report seeks to express why health decision-makers should be concerned about cloud technology, what they need to comprehend and be assured about urgently, and how to progress in a manner that prioritises the needs and preferences of patients and the public in health sector’s cloud integration,” Kalra added.
Cloud technology plays a pivotal role in enabling the data-centric strategy currently prevalent in healthcare. It has already demonstrated its potential in the field, offering key advantages like improved efficiency in patient-focused care, a population-oriented health approach, research that sparks innovation, and durable, resilient health systems.
However, the report highlights the industry’s lack of general awareness and comprehension of cloud technology. This lack of understanding leads to perceived risks, such as those related to privacy and security, which continue to hinder its broad adoption.
Suzanne Wait, Managing Director at The Health Policy Partnership, stated, “As both healthcare delivery and research have become increasingly data-intensive and collaborative, the process of gathering, merging, storing, analysing, and exchanging these data demands computational power, cybersecurity, and speed that far surpass typical onsite capacities, thus necessitating cloud technology. All stakeholders, not just IT departments, should enhance their understanding of ‘the cloud’ and ensure its proper and maximal usage across healthcare settings.”
The report emphasises the necessity for both patients and healthcare professionals to actively participate in discussions and policymaking concerning the technology. This involvement is key to ensuring that its implementation caters to their needs and incorporates their perspectives.
According to a recent report from Netskope Threat Labs, the healthcare sector has a comparatively low count of cloud malware downloads relative to other sectors. However, as cloud technology’s deployment expands, it is crucial for organisations to ensure they are adequately protected.
Read More